Having spent some time contemplating how image quality might be better reported to the community, I’ve decided to write a blog very explicitly dealing with my understanding of the main factors that will affect the quality of a given image within a study. I’ve then tried to investigate how we might very explicitly report this image, if we were reporting to an audience who demanded it. I’ve split these demands into what I think is ‘reasonable’ in terms of time and effort which would be taken to include in a paper’s supplementary materials, and those which may be asking a bit much, but I’ve put in for the sake of completeness.
Reasonable
1. Exposure/histograms + Camera settings
While camera settings are largely included in published studies, content detailing the exposure levels of the sensors are usually not. For my recent PICO presentation at EGU, I made an overlay of some basics we could include which dramatically impact the quality of an image, which include the camera settings and image histograms – this reported with the knowledge of the focal length (52mm) gives us an idea of how we might go about replicating the study.
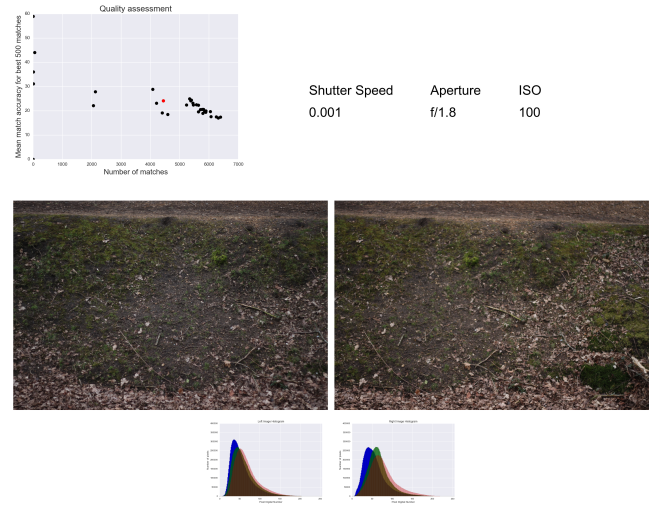
Template showing image settings and histograms
The settings effect the exposure, and the exposure has a histogram of a certain quality. While many natural images will have bimodal histograms, the distribution should give us some insight into image exposure. I’ve listed a few in the gallery below which are all ‘well exposed’, though have likely been post-processed. I would suggest as a start the mean and standard deviation of an image histogram would go some way into explaining image contents, and would be helpful alongside a representative image from a series. Glacial imaging is intuitively quite difficult due to the dynamic range required to capture a scene.
2. Diffraction level
I’ve written about diffraction limits (as well as MTF) in a previous post. I think many field surveyors using modern technology tend to steer clear of it as a concept, though I think it is important to bear in mind. If we look at, for example, the camera used for the above images (A Canon 500D), we can plot the various diffraction patterns we would expect at three different fstops as defined by the Rayleigh Criterion.
𝑑/2=1.22𝜆.𝑁 (We use 550nm (Green) for 𝜆)
Given many practitioners (particularly for low flying UAVs and oblique images) will need to stop up to ensure a sufficient depth of field, this is a very important consideration to make. While some level of diffraction is to be expected, and often gated using anti-aliasing filters which are in many consumer-grade cameras, reporting it is nonetheless important. The loss of detail is evident, though the effect on image-matching is yet to be quantified (To my knowledge) as it is so scene-dependant.

Courtesy of Phase One
3. Lens model/calibration
If used photogrammetrically, an idea of level of distortion helps give us intuition as to the quality of the optics in the system. This a standard step in orthorectification and could easily be reported. While generally left out, it may hint to reviewers/others as to how accurate the solution was, as we would expect the first two radial terms in Brown’s model (Here, k1 and k2) to be at least reasonably consistent between studies.
4. Expected Noise
If we are given the ISO at which imagery was acquired, we can get an idea of how much noise we might expect from parts of the imagery. The plot below is taken from Dxomark, and will give an expected SNR for the Canon 500D given various levels of incident light (as a factor of the maximum the sensor reads until saturated). Noise in db would put this more in line with lab testing, and move towards a scenario where studies using different sensors could be more easily standardised.

Taken from here
5. MTF of lense
Again, having written about this in a previous post, I can’t help but feel it’s more important than ever to report, or for field surveyors to at least be aware of. Whilst I won’t go into detail here, some sort of single number detailing the quality of the lens would be very useful for study comparison.
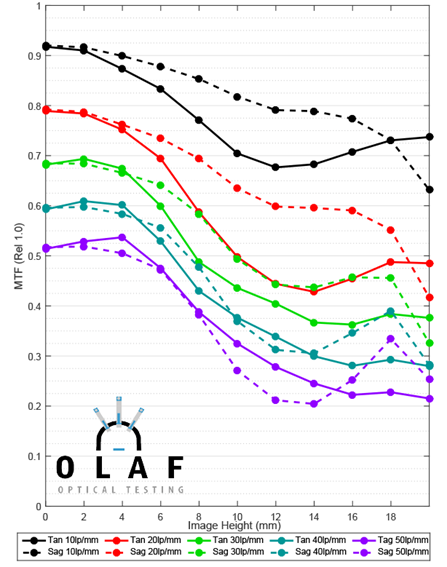
Taken from here
6. Expected blur (mainly UAVs)
Given how well studied blur is, and how far the development of detection algorithms have come, using the speed of the aircraft as well as the speed of lens to give an idea of how much blur would be expected is almost a requirement for study comparison.
While typically people will eyeball data and remove the blurry ones, studies have shown that even at low level blur, which could be difficult to percieve, can have destructive qualities on image-matching.
Not so reasonable
1. Incident light level
This is unreasonable, but a sky facing camera of similar specifications in order to get an idea of incident light would be extremely useful for exposure control/looking at BRDF of the surface. I imagine something not unlike the AERONET sun photometer network, in order to do some correction for incident light. Considering the wealth of information we have into atmospheric conditions given the number of satellites in orbit, leveraging this against what we do on the ground seems like it should be worthwhile.
2. Spectral calibration
One that I’ve harped on about before in this post, a spectral calibration would allow correct colour balance between images on the ground/satellite, allow us to optimise contrast balancing depending on the task being addressed and undoubtedly offer a level of repeatability we currently don’t have. Unfortunately it doesn’t seem like this will happen soon, as creating suitable control conditions is extremely difficult, and short of using an integrating sphere I don’t know how it could credibly be achieved.
Conclusion
I’ve wrapped this whole post into a TL;DR for those looking for a quick fix. All of what’s listed below is largely obtainable from online sources and would go a long way into standardizing the reporting format of images taken from consumer-grade cameras for metric use.
Theme | What to report |
---|---|
Exposure level/Camera settings | ISO, Aperture, Shutter Speed, Focal length, mean and standard deviation of representative histogram |
Diffraction level | Sensor size, aperture, number of pixels |
Lens model | Radial distortion correction coefficients |
Expected noise | Noise in db |
Lens MTF | Expected loss of contrast based on image features |
Expected blur | Blur in pixels given survey design |